Inteligência Artificial tem melhorado ao ponto que imagens e vídeos gerados por IA são muito convincentes e indistinguíveis da realidade. Entramos na era em que IA pode se tornar uma poderosa fonte de inverdades, fake news etc.
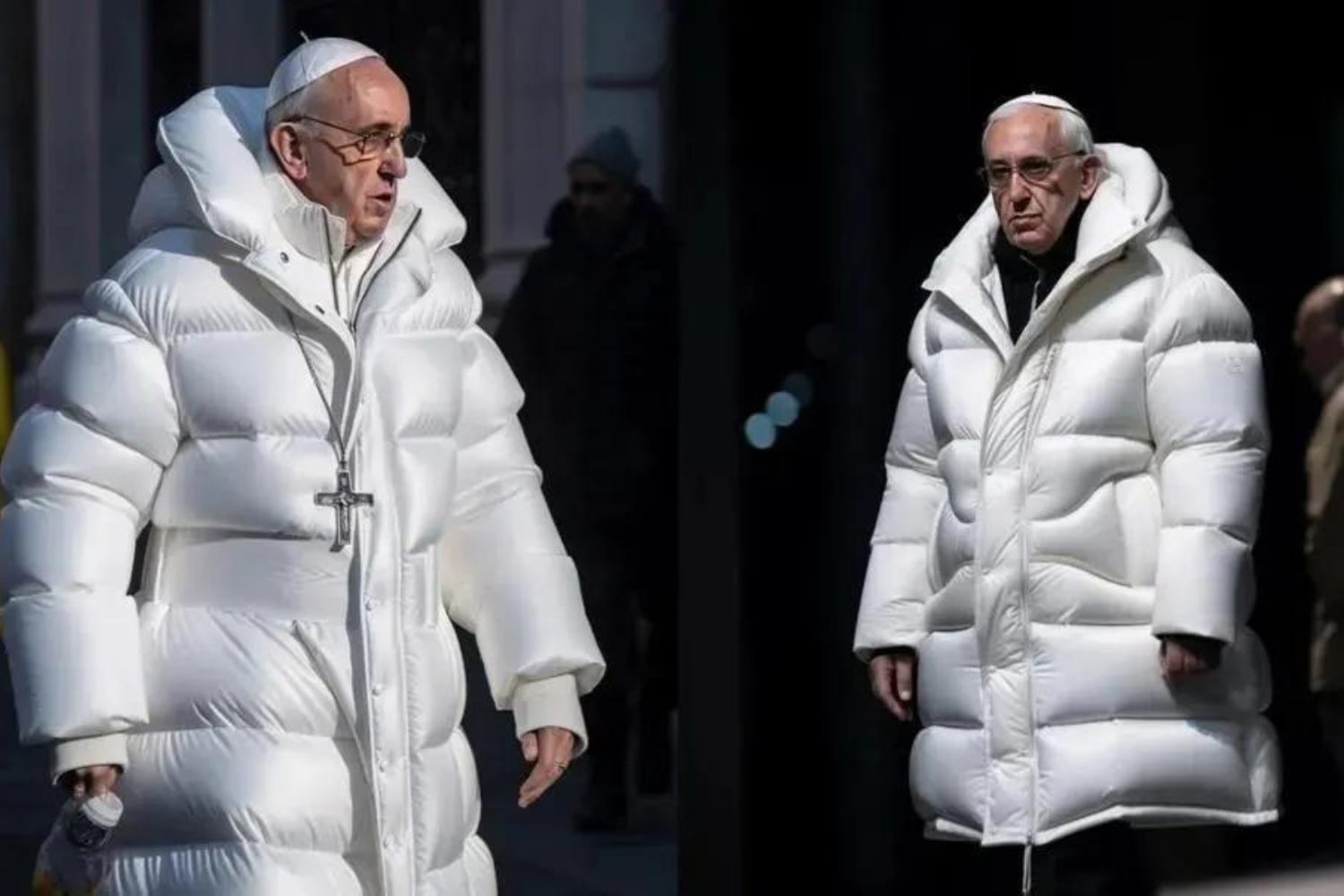
Inteligência Artificial tem melhorado ao ponto que imagens e vídeos gerados por IA são muito convincentes e indistinguíveis da realidade. Entramos na era em que IA pode se tornar uma poderosa fonte de inverdades, fake news etc.
The new iOS 18.4 has an unbelievably wonderful, unprecedented — and somewhat hidden — feature.
Ambient Music!
There are four buttons you can activate in the 🎛️ Control Center that provide endless ambient music in four styles and for four purposes:
Read MoreChamando todos os cientistas de dados, sobretudo os que lidam com séries temporais, como eu, para ver um experimento.
Read MoreThere is this study (arXiv:2308.02312v3) about ChatGPT as a software coding assistant when compared to Stack Overflow. I write code every day and although I have used ChatGPT in the past for this purpose, I have a bias towards believing that humans answering on Stack Overflow would be better. Apparently the study findings below confirm my beliefs. But maybe, just maybe, LLM tools can be specialized or improved to be better than the amazing Stack Overflow. Anyway, I would never rely on LLM for a complete app refactor or overall design, since it requires architectural knowledge, real life experience, lessons learned, context around and strategic vision, multidisciplinary features of grown up, real, human, experienced software engineers.
The findings:
Read MoreI’m doing Machine Learning Industrialization for more than 2 years and I’m thrilled to see it featured by McKinsey as top 2 in its 2023 tech trends!
Read MoreThis is often the outcome when AI projects lack Machine Learning Engineers and rely solely on Data Scientists.
Read MoreThis diagram highlights the importance of Machine Learning Engineering for Data/AI projects and the community. And it doesn’t even show one of my favorite topics: software design patterns, an outrageously important subject that helps with code maintainership, extensibility, standards, organization, beauty, which in turns help with (much) higher productivity of Data professionals.
Read MoreYes, Data Scientists should develop their software engineering skills. Let me react to a LinkedIn post by Neil Leiser.
But Data Scientists can’t do it alone, or by themselves. Read on.
I see that software engineering, IT architecture is a touchy subject amongst even the best data scientists, usually because they came from other knowledge domains as economy, statistics, pure math, physics, biology etc. This is a normal evolution. Data Science demands a wide broad skill set, sometimes too wide and too broad. Data Scientists need to handle Docker and HTTP APIs along with outliers, RMSE, ROC curves and Gaussian distributions. Go figure…
ML engineers — usually folks that have more software engineering background — should help here.
But the most important thing ➔ it is the mission of the CDO, tech lead or CTO with strategic vision to clearly detect these gaps and design a roadmap to handle them, not just with conventional training but also encouraging mixed squads whose members will exchange skills and knowledge, leveraging multi-disciplinar environments where everybody grows together.
Related posts:
This is what GPT “knows” about me. More precisely, this is the sequence of words GPT generates when asked with that specific prompt.
First paragraph is 100% correct.
Second is kind of 50% (in)correct and outdated. I do Fedora, not Debian nor Ubuntu, I’ve contributed to several FOSS projects, but never to Apache HTTPD, and I did work for IBM, but never to Red Hat.
Third paragraph he completely confused me with one of my relatives that have same last name but different first name.
Also, I think GPT would have a different perspective about me if blog posts in social media, such as Facebook, would be part of its training dataset. But it can’t because Meta won’t allow open access to their platform even if I post openly there.
Analysts inform, explain and visualize DATA THAT EXISTS in order to help business executives make strategic decisions. Thus, data analysts live in business meetings, talk to a lot of people and create data visualizations to help others understand what is going on. Tools: SQL, BI, spreadsheets, PowerPoint.
Scientists infer and calculate INFORMATION THAT STILL DOESN’T EXIST, such as the future, usually in order to optimize each and every business transaction. Example: if you like this one product, you might also like that other product. Example: according to data from surroundings, this house price should be around $X. Example: I learned how cars look like, so there is 98% chance there is a car in this photo. Thus, they create or improve digital products using machine learning and applied statistics. To create such improved user experiences, first data scientists use advanced exploratory data analysis techniques, create data visualization only for themselves, only for their better comprehension of what is going on. Tools: SQL, Pandas, math and statistics, git, programing, containers, Linux.
Data analysts tend to have a more glamorous job, while data scientists job is more hard skills oriented. Both need to work with large amounts of information, such as tables with millions or billions of data points.
There is also the Data Engineer role, which is as important as these other data professions, and focused on data availability, consistency and performance.
Inspired by Gerson Lerner’s post, I thought I should give my take on the subject too.
Streamlit (streamlit.io) is a lovely Python module that helps data scientists build interactive dataviz apps.
Use it when a BI is overkill — as this Streamlit dashboard that I wrote to manage my personal investments —, or where there is no BI, such as very small companies. Or where there is no interactive app developers to create a native app.
Read MoreOpen Data Science Conference 2022 has happened in Boston this week. Conference featured panels, workshops, presentations and a vendor expo. I attended the 3 days and here are some impressions.
Que satisfação ver meus alunos da Digital House ingressando em novas empresas, em cargos de dados.
Como eu vivo dizendo a eles, Dados é uma oportunidade continental, equivalente a descoberta do Novo Mundo em 1492. E é também como sexo de adolescente: todo mundo diz que está fazendo, mas na verdade ninguém, praticamente ninguém mesmo, está fazendo direito.
E é essa geração de profissionais de dados que farão acontecer.
Mãos à obra!
I’ve seen companies saying they have Big Data because they implemented Hadoop or a data lake and maybe Spark.
That’s just wrong.
Big Data, or more precisely, to be Data Driven, is a state where the data a company produces can be reused, as soon as possible, to optimize itself. And there are many ways to reuse data: all meetings and decisions happen with abundance of data, or recently generated data instantly feeds machine learning algorithms to optimize transactions, just to name a few situations.
To be Driven by Data is part culture and part infrastructure. On the infrastructure side, IT teams still struggle with limited visions about how data should flow pervasively and how access should be granted. They fear about security and performance while they should fear of missing out the data opportunity.
Data Streaming is a breakthrough recent technology that is here to help with more fluent data access. For an agile and effective data architecture, Data Streaming is much more strategic and important than just a bigger data warehouse because it is the component that can unleash your data and finally make it useful.
Apache Spark is like Python’s Pandas and is like SQL databases. It can manipulate datasets, filter, integrate, transform.
But Spark was designed from scratch with horizontal scalability and parallelism in mind, which makes it capable of handling datasets with billions or even unknown number of rows — even if a bit less flexible than Pandas.
This is not new in the industry. Enterprise editions of commercial SQL databases are parallel and scalable since a very long time, being also very expensive in all levels of the stack: service/support, software and hardware.
But Spark is free software. And can use Hadoop — also a free software — as scalable and highly available storage, on cheap commodity hardware. In addition, it has a vibrant community and a democratic ecosystem of services and support.
As with all Open Source, Apache Spark changes the economic landscape of massive data processing systems market, taking money out of a few proprietary HW and SW vendors and pulverizing it locally on people and support.
2020 list of desired hard skills for data professionals. From the most essential to the more difficult ones.
Please remember this list has only hard skills. Ethics, domain and industry knowledge, communication are very important soft skills that won’t fit in this list.
Generally speaking, beginning of the list is where Data Analysts are (up to ≈11). Data Engineers get up to the middle of list (up to ≈18). And Scientists get all the list.
There is also the following graph that I’ve produced:
Se sua missão é entregar dados a usuários, soluções de Business Intelligence open source baseadas em Python emergem como opções muito atrativas frente aos proprietários MicroStrategy, PowerBI, Cognos, Google Data Studio, Tableau etc.
Read MoreA profissão de Cientista de Dados ganhou notoriedade e eminência nos últimos anos. Uma figura que tem saído do círculo hipster das startups e invadindo empresas de todos os tamanhos. Até os mais tradicionais executivos já compreendem que, tendo seu negócio entrando na era da informação e se tornado uma usina de dados, há que se lançar mão de profissionais especializados, que saibam como tirar proveito de todos esses dados.
Mas será que está claramente compreendido qual é o papel do Cientista de Dados? Sabe-se o que esperar dele? Consegue-se usufruir de todo o seu potencial?
Enquanto os outros profissionais da área de dados, se aproximam ou mais do TI (como o engenheiro de dados) ou mais do negócio (como o analista de dados), o Cientista trabalha o tempo todo junto às duas áreas.
Enquanto os outros profissionais de dados tratam da curadoria, performance, qualidade, apresentação de informações que existem, o foco principal do Cientista de Dados é calcular tendências e inferir dados que ainda não existem.
O Cientista de Dados está próximo do negócio. Entende sua semântica, desafios e necessidades. E tem plena consciência das informações que tem a sua disposição, sejam privadas ou públicas. E quais novas informações pode derivar delas. Navegar nesses dois mundos — dados e negócios — permite ao Cientista de Dados fazer melhores perguntas e já trazer respostas sobre o negócio — respostas que são os dados que ainda não existem. Por exemplo:
Repare que muitas dessas perguntas estão relacionadas ao futuro, a informações que não existem ainda. Cientistas de Dados conseguem prever o futuro — ou a probabilidades de eventos acontecerem — e é por isso que são verdadeiros oráculos.
Essa capacidade do Cientista de Dados também coloca-o na posição de literalmente poder calcular a chance de sucesso de uma determinada iniciativa de negócio.
Em 2013, Gartner explicou ao mundo o nexo das novas forças de TI, onde Dados, Mobilidade e Redes Sociais têm um papel determinante para o sucesso de qualquer empresa que quer se manter moderna.
Fica claro que a Mobilidade é a rota para uma organização chegar nas pessoas, sejam elas clientes ou colaboradores. Alavancar também o poder colaborativo das Redes Sociais para fomentar uso e fazer os Dados circularem. No começo dessa era, houve uma corrida para empresas criarem suas apps, que nada mais eram do que o catálogo digital de seus produtos, ou seu site institucional no smartphone, ou até mesmo prover diretamente os dados a seus usuários, como seu saldo ou extrato. Pouca novidade até aqui. Mas a era da Mobilidade representa o momento histórico em que organizações passam a estar constantemente ao lado de seus clientes e colaboradores, quando acordam, quando trabalham, quando almoçam, quando vão dormir. Mais ainda: se o usuário der permissão para a app, ela poderá chamá-lo para interagir ou entregar uma novidade, através das notificações que aparecem na tela.
Mas qual informação a app vai entregar proativamente ao usuário? Quando? Onde? É o Cientista de Dados que tem a responsabilidade de fazer a ponte entre os dados virgens e a app do usuário, no sentido de entregar a informação certa na hora e no lugar adequado, com o objetivo de tocar o coração de quem usa.
As possibilidades são infinitas. Um exemplo no varejo é determinar quais são os clientes que devem receber uma notificação às 11:45 da manhã informando que já está disponível na loja nas redondezas onde ele costuma almoçar um produto que vai lhes interessar.
Um outro campo interessantíssimo para o Cientista de Dados é próximo ao fenômeno da Internet das Coisas, onde sensores coletam constantemente dados do ambiente a sua volta. Inclui-se aqui também sensores biométricos, que medem indicadores do corpo humano, como características do sono, pressão sangüínea, alimentação etc. O Cientista de Dados é a figura que agrega esses dados — que isoladamente têm baixa significância — encontra correlações pouco óbvias e é capaz de re-injetá-los no processo do negócio para assim transformá-lo, otimizando ações que outrora eram reativas, em proativas.
Dizem que o Cientista de Dados é um estatístico que conhece mais ferramentas computacionais que um estatístico médio. Ou também um programador que conhece mais estatística que um programador médio.
Base sólida em matemática, probabilidade e pensamento estatístico, boas noções de econometria, bons conhecimentos em ferramentas computacionais como machine learning, fazem um Cientista de Dados ser prático e mão-na-massa. Multidisciplinaridade e traquejo para circular nos corredores do negócio são extremamente importantes também. Apesar de seu pé na programação, o estereótipo engraçado do programador que vive a base de pizza e cafeina não combina muito bem com o Cientista de Dados.
Claro, há bons profissionais que não programam ou deixaram de ser mão-na-massa. Estes acabam atuando coma uma espécie de CDO (chief digital officer), com o adicional de que têm experiências mais concretas sobre onde pode-se chegar com os dados. Mesmo assim, ele terá que lançar mão de outros Cientistas de Dados que programam e são mais mão-na-massa.
O Cientista de Dados geralmente programa em Python, usa Jupyter e é bom conhecedor do ferramental de visualização e gráficos dessa linguagem, bem como as de machine learning e inferência estatística. SciKit Learn, StatsModel, Pandas, Seaborn, XGBoost, Shapley é o arroz-com-feijão deste profissional.
Respire fundo e arregace as mangas caso escolha ser um Cientista de Dados. A gama de disciplinas exigidas é larga. Vai de matemática, programação, TI, até o negócio, cultura e intuição sobre o mundo a sua volta. Prepare-se para programar com ferramentas avançadas e que sempre estarão em voga. Prepare-se para conhecer o negócio em nível matemático e também multicultural e multidisciplinar.
Mas chegando lá, você será singular em sua roda. Terá uma posição sob os holofotes em seu meio profissional, devido a sua bagagem de conhecimentos e capacidade de transformação.
Para se apreciar uma bela vista, é necessário escalar uma alta montanha. E o Cientista de Dados é o que está em seu topo.
Se você está numa encruzilhada para escolher uma linguagem de computador para aprender a programar, escolha Python.
Read More